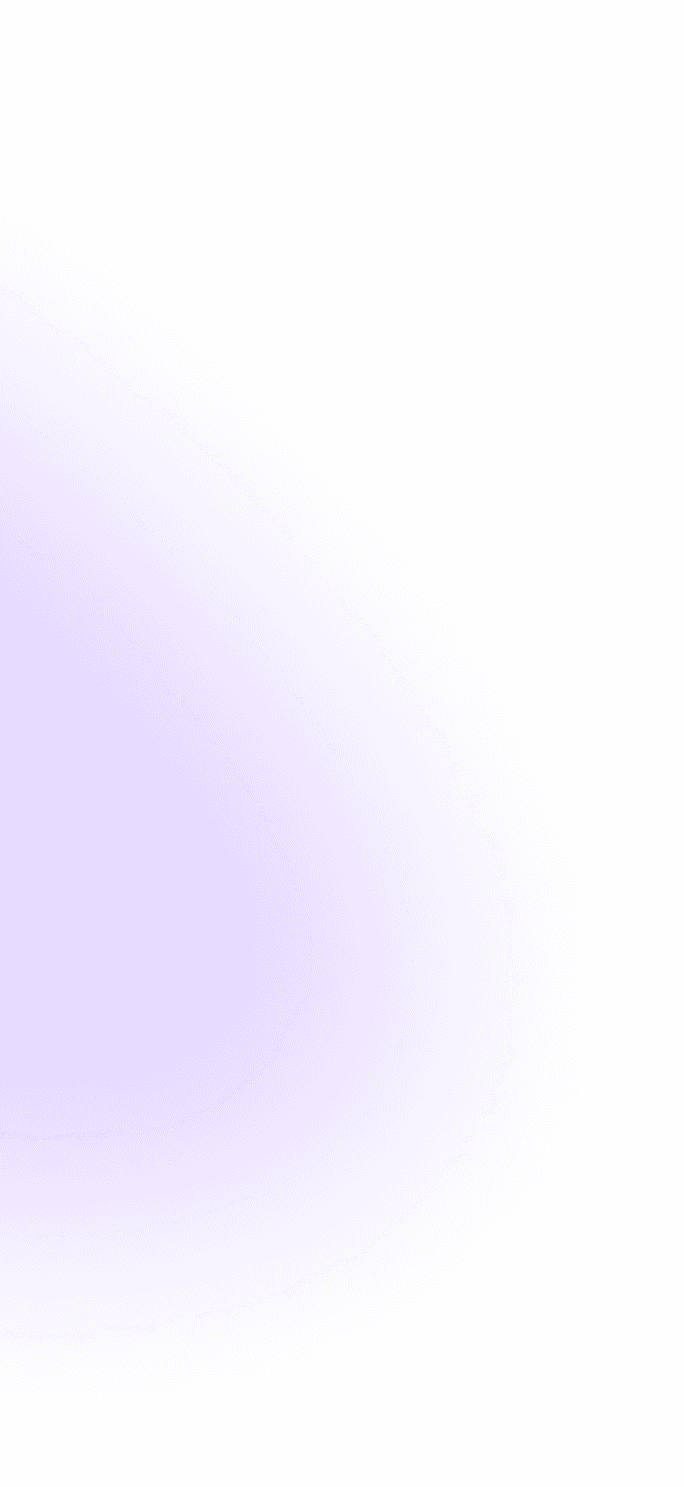
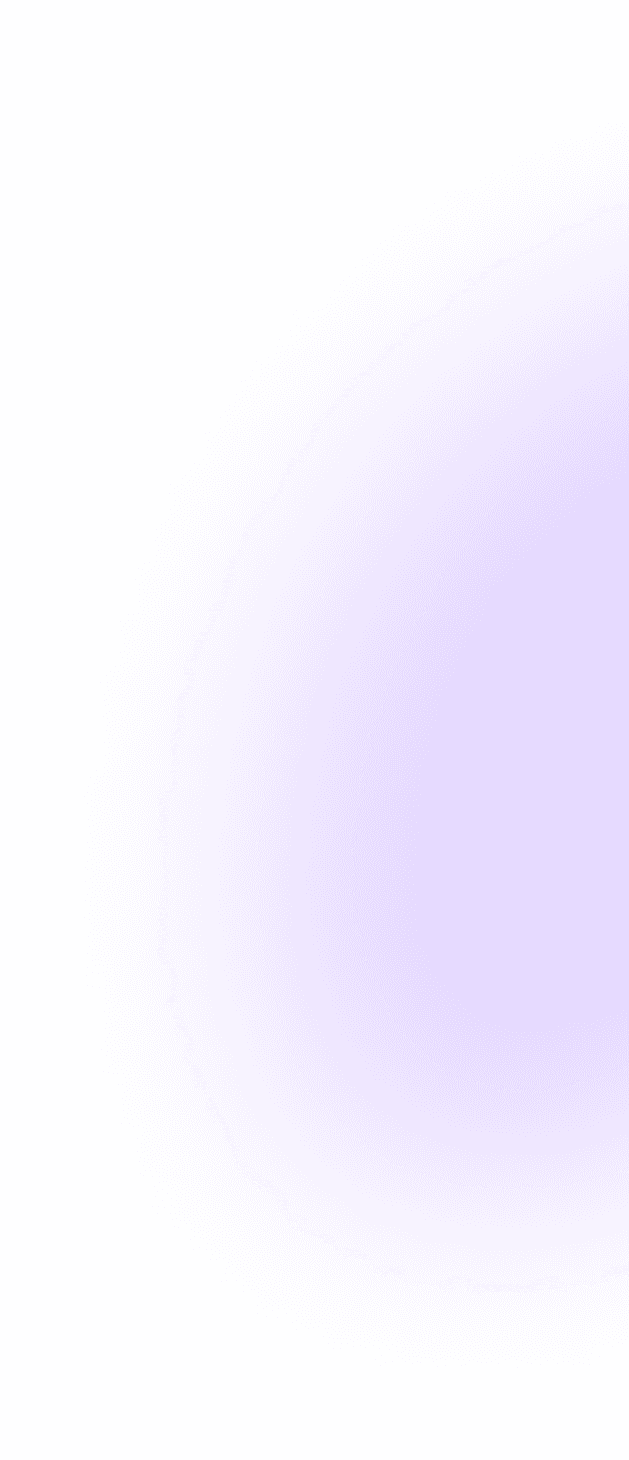
Selecting an AI-Driven Quantitative Approach to Algorithmic Investing
Mar 26 | 5 Mins MIN | Institutional investment
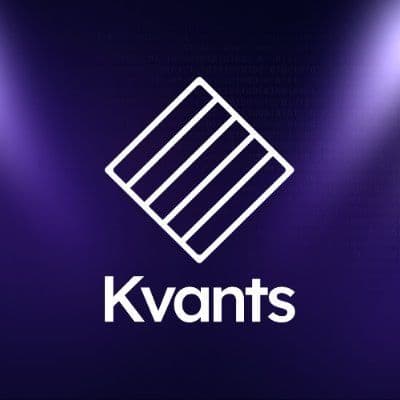
By
Kvants Team
Key Highlights
Don’t Just Look at Returns—Understand the Metrics
A strategy’s return profile alone doesn’t tell the full story. Assess Sharpe ratios, drawdowns, win rates, and consistency across market cycles.
The Importance of Track Record and Transparency
Established strategies with live performance data and clear methodology provide greater confidence. Understand how the algorithm works at a structural level.
Match Strategy to Your Risk Appetite
Aggressive, balanced, and conservative strategies behave differently. Select one that aligns with your tolerance for volatility and drawdowns.
AI Should Be Adaptive, Not Static
True AI evolves in real time. It should continuously learn from new data and adapt to changing market regimes, not follow static rules.
Diversification Through Multi-Strategy Exposure
Markets are unpredictable. Diversified exposure across assets, strategy types, and timeframes offers better risk-adjusted returns.
In today’s fast-moving markets, relying solely on human intuition and discretionary decision-making has become increasingly limiting. Data is abundant, markets are increasingly algorithmic, and the pace of change demands a more sophisticated approach to investing. This is where AI-driven quantitative trading strategies come in—offering a combination of statistical modeling, automation, and machine learning to deliver intelligent, rules-based execution across a range of assets. However, selecting the right AI-driven strategy requires more than just choosing the one with the highest historical return. Investors must assess the logic behind the strategy, its performance consistency, adaptability to market conditions, and risk management mechanisms. Whether you're allocating a portion of your portfolio to a systematic fund or accessing strategies via a digital platform, here’s how to evaluate and select the right AI-powered quant strategy.
Understanding the Foundations of AI-Driven Quant Strategies
AI-driven quantitative strategies are rooted in data. They use mathematical models to identify trading opportunities based on statistical relationships, historical price patterns, or real-time market behavior. These systems are designed to minimize human bias and respond dynamically to changes in volatility, momentum, volume, and other market indicators. What sets AI-driven strategies apart from traditional quantitative models is their ability to learn and evolve. Rather than relying on fixed rule sets, machine learning algorithms can adjust their parameters over time, optimizing themselves based on performance feedback loops or changes in market structure. This makes them especially valuable in complex, fragmented, or volatile environments like cryptocurrency and FX.
Key Considerations When Evaluating a Strategy
The promise of AI can be compelling—but not all strategies labeled “AI-powered” are truly intelligent or adaptive. When assessing any quant strategy, investors should focus on the following dimensions:
Don’t Just Look at Returns—Understand the Metrics
Raw performance numbers can be misleading and should never be evaluated in isolation. A strategy boasting a 200% annualized return may also have endured a 70% drawdown or relied heavily on excessive leverage—factors that can dramatically alter its real-world appeal. Instead of focusing solely on returns, investors should prioritize risk-adjusted metrics that offer a more comprehensive view of performance relative to volatility and downside risk.
The Sharpe ratio is one such measure, indicating the amount of excess return generated per unit of risk; a Sharpe above 1.0 is generally acceptable, while anything above 2.0 signals strong risk efficiency.
Maximum drawdown helps illustrate the worst-case decline the strategy has experienced from peak to trough—critical for setting expectations during market stress. Additional metrics like win rate and profit factor shed light on the strategy’s consistency and profitability per trade.
Perhaps most importantly, investors should evaluate how the strategy performs across different market regimes, rather than excelling in just one particular phase. A well-balanced, adaptable strategy should demonstrate resilience and reliability, not just isolated success.
The Importance of Track Record and Transparency
The more transparent a strategy is, the more trust it earns from investors. While few platforms or managers are expected to reveal the full details of their algorithmic logic, a credible strategy should at the very least provide clarity around its methodology, historical behavior, and how it adapts to changing market conditions. Key questions to consider include whether the strategy has a live trading history or relies solely on backtests, how long it has been operating in real-time conditions, and whether its results are independently verified—either through on-chain records or third-party tracking tools. It’s important to note that newer strategies with limited live performance aren't inherently flawed, but they do carry more uncertainty and should be assessed with added scrutiny. This is especially true for AI models, where the risk of overfitting is high; a model that excels on historical data but underperforms in live trading may not have been adequately stress-tested.
Match Strategy to Your Risk Appetite
A well-designed AI strategy is only effective if it aligns with the investor’s specific goals and risk tolerance. One of the most common mistakes is selecting a model purely based on strong past performance—often in a specific market phase—without considering how it fits into a broader investment strategy or time horizon. AI quant strategies typically fall into three categories. Aggressive strategies aim for high returns but tend to carry greater exposure to volatility and deeper drawdowns. Balanced strategies seek to smooth performance by combining approaches such as trend-following, mean reversion, or multi-asset allocation. Conservative strategies, on the other hand, prioritize capital preservation and tend to operate with tighter risk controls, often focusing on stable asset pairs or arbitrage opportunities. Understanding where a strategy fits along this spectrum is essential for proper portfolio construction and long-term performance consistency.
AI Should Be Adaptive, Not Static
One of the core advantages of AI models is their ability to adapt—but not all so-called AI strategies fully capitalize on this. In many cases, platforms label simple automated scripts as “AI” when they are, in reality, static rule-based bots lacking true intelligence. A genuine AI-driven system should learn from evolving market conditions, adjust its parameters based on recent outcomes, and continuously optimize itself using real-time feedback. Without this adaptability, strategies risk becoming obsolete as market dynamics shift. In fast-moving or volatile environments, model degradation is a significant concern. Static strategies that once performed well may fail when faced with new patterns or behaviors. For this reason, investors should seek out strategies that incorporate continuous learning and are designed to operate across multiple assets and market regimes.
Diversification Through Multi-Strategy Exposure
Markets behave differently across timeframes and macro environments, and no single strategy is equipped to perform consistently well under all conditions. This is where multi-strategy investing proves valuable. By allocating capital across models that are designed to thrive in different scenarios, and that are ideally uncorrelated, investors can build a more resilient portfolio. For instance, momentum strategies tend to perform well in trending markets, capturing sustained directional moves. Arbitrage strategies, on the other hand, often excel in sideways or inefficient markets by exploiting temporary pricing discrepancies. Meanwhile, volatility strategies are positioned to benefit from sharp price movements and market uncertainty. When these approaches are combined, they can help offset one another’s weaknesses, reducing drawdowns and smoothing out returns over time. This framework is particularly effective in crypto and emerging markets, where volatility is elevated and regime changes occur frequently, making a single-strategy approach riskier and more prone to underperformance.
Balancing Automation with Oversight
Even the most sophisticated AI strategy benefits from human oversight. Investors should have access to clear reporting, control mechanisms (e.g., pause or reallocate capital), and updates on performance and methodology shifts. Regularly reviewing how the strategy adapts to changing conditions—and being able to intervene if needed—is part of responsible portfolio management. This is especially relevant in highly automated environments, where strategy drift or technical issues could lead to compounding errors if left unchecked.
Final Thoughts
AI-driven quantitative strategies represent a fundamental shift in how capital is allocated. For institutional and sophisticated investors, they offer speed, discipline, and the ability to uncover opportunities far beyond human reach. But with this power comes responsibility. Choosing the right strategy means going beyond performance charts—it means understanding the underlying structure, risk behavior, adaptability, and transparency of the system. The best investors will not just ask “what’s the return?” but also “how is that return being generated?”, “what risks are being taken?”, and “how does this model behave in uncertainty?” Whether accessed through a platform, fund, or built in-house, the role of AI in trading is only expanding. But like any tool, its effectiveness depends entirely on how—and where—it’s used.

Read more
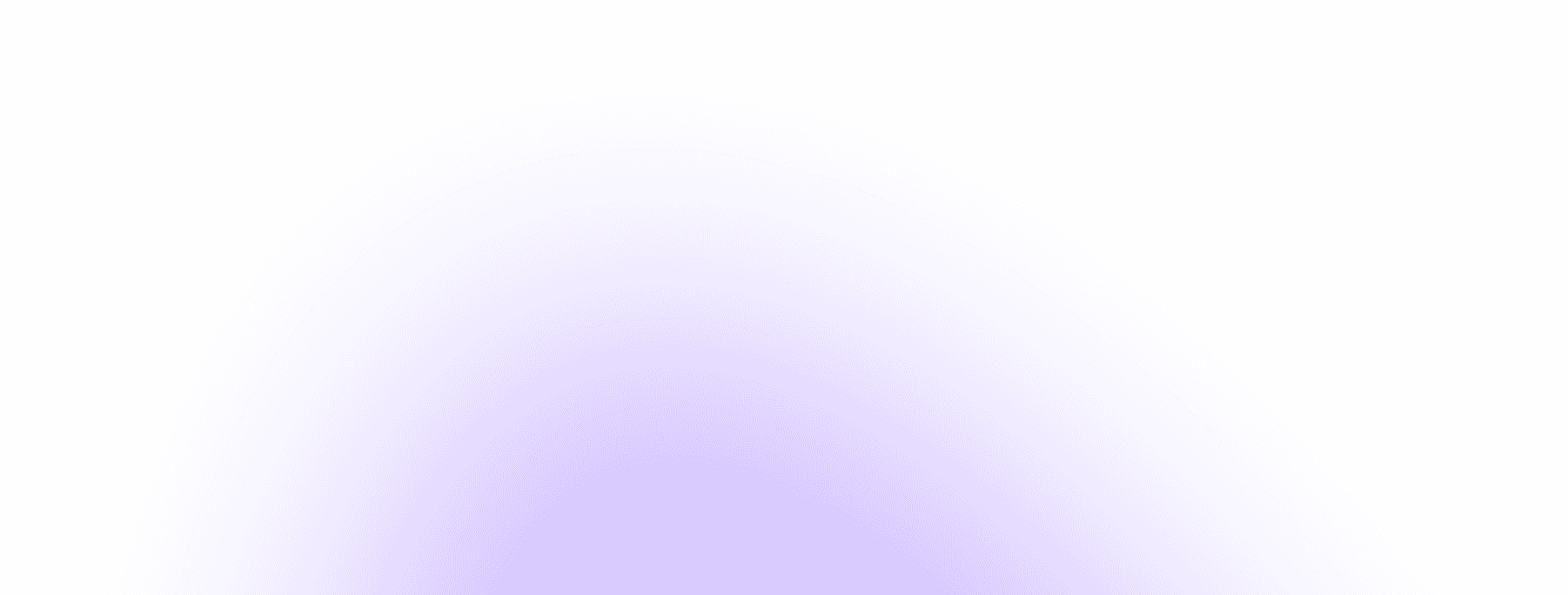